In just two months, ChatGPT reached 100 million users, outpacing TikTok and WhatsApp. This shows AI’s growing popularity, especially versatile generative AI. In 2024, generative AI’s influence in tech and finance is undeniable. This article looks at key generative AI trends in finance for 2024.
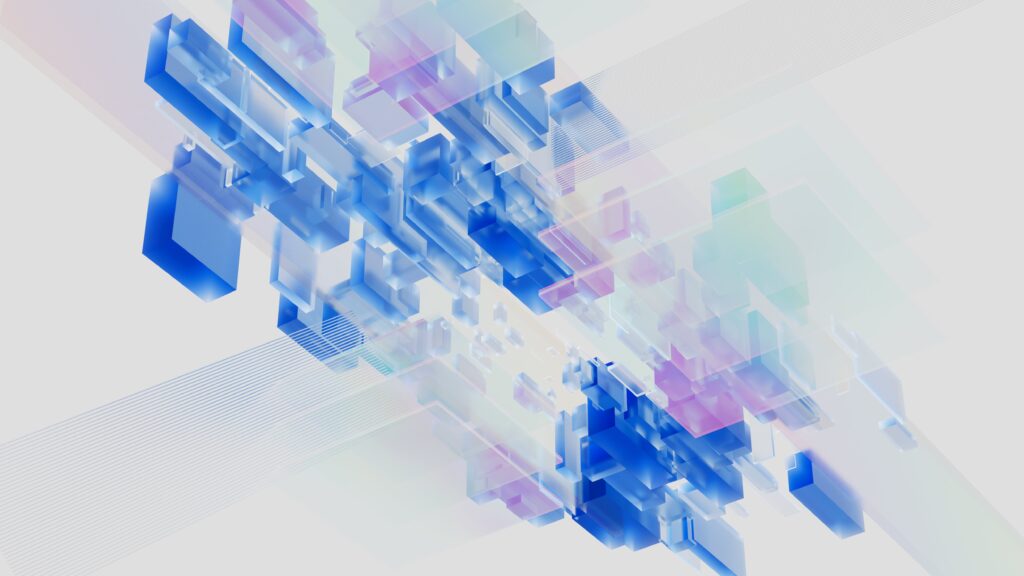
Artificial Intelligence (AI) is a broad field that aims to make machines act like humans, including areas like Machine Learning (ML) and Generative AI (Gen AI or GAI). ML, part of AI, helps machines learn from data and make choices. GAI, which grew from ML, is about creating new data. GAI is all about generating something new: images, text, even video. There are two most widely used generative AI models and they are the following: Generative Adversarial Networks (GANs) – are a subset of GAI and are technologies that can create visual and multimedia artefacts from both imagery and textual input data1. Large Language Models (LLMs) – are a subset of GAI. LLMs are technologies such as Generative Pre-Trained (GPT)2 language models that can use information gathered on the Internet to create textual content from website articles to press releases to whitepapers3. Artificial General Intelligence (AGI): AGI is a theoretical concept that represents a level of AI where machines can perform any intellectual task that a human can do or even perform beyond human-level intelligence across various areas. It is commonly mistakens with FA4. |
Why focus on this? In the swift-paced finance world, choosing the right tech is crucial. Too many options can lead to confusion. A single, well-chosen use case can help us get started with generative AI.
Before we dive deeper into the use cases, let’s think about the general gen AI benefits for business. We could list the following examples:
- Conversational Customer Service: Gen AI turns online chats with customers into smooth, helpful conversations. It can automatically answer questions and provide information, making customer service faster and friendlier.
- Easy Access to Complex Data: Gen AI helps businesses easily understand and use large amounts of data. It can find and suggest products, help with searches within the company, and make work processes more efficient.
- Quick Content Creation: Gen AI allows businesses to create various types of content quickly, like marketing materials, documents, or even software code, saving time and boosting creativity.
Some use cases here might be ones you know. Gen AI hasn’t just brought new things to the financial sector; it’s mainly made existing ones better. Traditional AI, like Apple’s Siri or Google search, follows set rules for tasks. Gen AI is different. It learns from lots of data, not just set rules. This way, it keeps improving and can do things as well as, or sometimes even better than humans.
Fraud Detection Evolves with gen AI
In finance, it’s very important to catch fraud fast because fraud methods keep changing. Traditional Machine Learning (ML) systems, which rely on old data, often miss new kinds of fraud. Generative AI, including a type called Generative Adversarial Networks (GANs), is making a big difference here.
Gen AI uses advanced but easy-to-understand methods to look at data right away. It uses deep learning and neural networks to do this. Gen AI can spot new and hard-to-see fraud patterns that older systems can’t. GANs, an essential part of Gen AI, have two parts: the generator and the discriminator. The generator is responsible for creating new content such as data, while the discriminator evaluates the quality of the generated content by comparing it to the real data. What does this exactly look like in a fraud detection case? The generator creates artificial transactions, while the discriminator evaluates their authenticity5. This teamwork helps GANs learn fast and adapt, so they’re really good at finding complex fraud.
Gen AI, especially with GANs, gets better over time as it learns from new data. This means it can defend against fraud in a more up-to-date way than traditional ML. Banks and financial companies that use Gen AI are better at protecting their customers and their business. They stay ahead in stopping fraud.
For example, in this Workshop: Credit card fraud detection with AI/ML (Feb 16, 2022), we explore how AI/ML can speed up credit card fraud resolution.
24/7 Customer Service with Gen AI
Gen AI is changing how banks help their customers, focusing on fast and smooth service. In today’s online banking world, customers want quick help for things like credit card issues, bill payments, or starting new accounts. Gen AI makes this quick help possible, reducing the need for people to step in and offering help right away.
This change is thanks to tech like Large Language Models (LLMs), which are behind tools like IBM’s watsonx Assistant. These models create text that sounds like a human, so you can “talk” to them and get answers. This leads to what you can call conversational banking, where talking to the bank feels more natural and human. These tools use a lot of banking data and AI to understand what customers are asking and give correct, fast answers.
The result is customer service that’s available all the time, not just efficient but also very tailored to each person. Banks using gen AI can better meet their customers’ changing needs, providing service that fits the quick-moving world of finance today.
AI Chatbots: Personalized Financial Advice
Machine Learning (ML) tools have become integral in banking, especially in identifying which sales or marketing strategies might resonate with different customer segments based on their transaction history and profile data. However, the challenge often lies in rapidly deploying these insights. For instance, creating personalized financial advice messages within banking apps requires significant effort and resources. This is where generative AI is making a substantial impact.
Robo-advisors in wealth management are giving way to gen AI chatbots6. In 2024, chatbots are becoming smarter, thanks to advancements in LLMs. They’re moving beyond scripted responses to engaging in natural conversations, possibly offering personalized financial advice.
IBM’s watsonx Assistant is a great example of this new tech. It uses advanced AI to understand customer data, making banking more relevant to each person (See graph 1.). It gives useful suggestions and advice, which makes banking easier and improves the customer experience. This way, customers get help that feels more personal and useful.
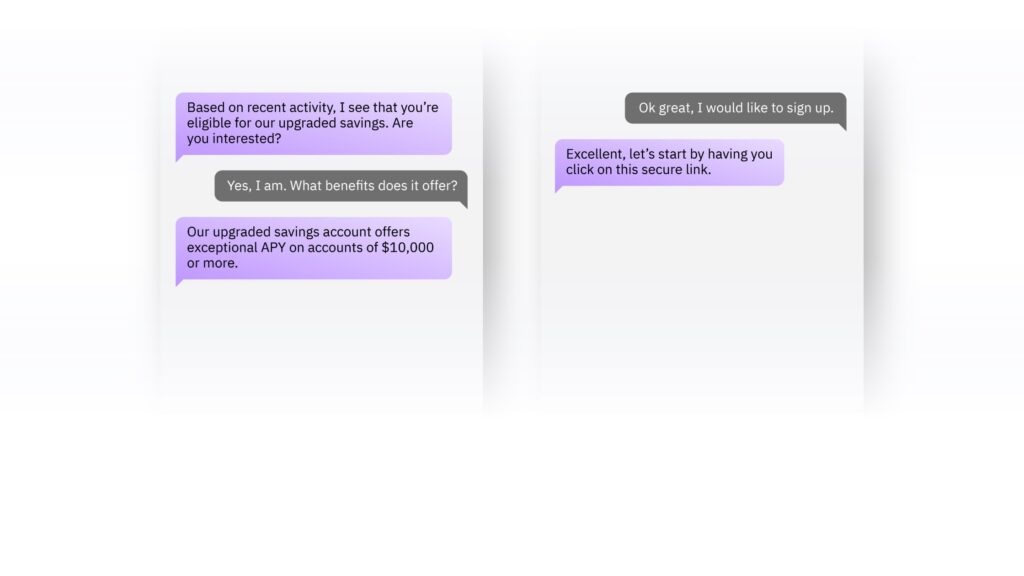
Revolutionizing Cybersecurity with Gen AI
OpenShift AI provides a secure, enterprise-grade AI platform
In banking, gen AI is crucial for risk management and cybersecurity. With cyber threats increasing, as indicated by a 48% rise in attacks reported by ISACA in 20237 firms like Red Hat are providing security and compliance solutions that can be a solid and secure foundation for gen AI tools. This shift is transforming how banks defend against cyber threats.
Check how Red Hat sees AI in Banking!
Just as generative AI algorithms like GANs are used to create synthetic data resembling real fraudulent transactions, they can also be employed in cybersecurity. These algorithms can generate data that mimics cybersecurity threats, such as malware, phishing attempts, or unauthorized access attempts.David Reber Jr., Chief Security Officer at NVIDIA, highlights how banks can use data from multiple sources and machine learning algorithms to detect malicious activities that might be missed by human analysts8. Synthetic data improves financial data by adding examples of rare situations that are important for training gen AI models. This makes it easier for the gen AI to learn patterns in data and deal with big cybersecurity problems. For example, gen AI can make a lot of training data for phishing, creating very realistic texts and email attachments. This helps security experts teach models to spot real fake emails better, lowering the chance of mistakes and improving the fight against complex attacks.
Reimagine your bank with Red Hat
What are the potential risks of generative AI?
Using gen AI in banking has advantages but also comes with risks. Protecting customer data is a big concern because gen AI relies on sensitive information. Sometimes, gen AI can make mistakes or show bias based on old data, which may lead to unfair decisions.
Moreover, there’s a risk that cybercriminals could use gen AI to deceive people with fake emails or messages. To manage these risks, banks start by using gen AI for small, internal tasks to ensure security and compliance.
Virtual gen AI chatbots acting as financial advisors in Europe face challenges like strict data protection laws (like GDPR), the complexity of providing personalized advice, and customer preference for human interaction. Technical limitations, ethical concerns (like unfair bias), and cybersecurity risks also make it harder to use them widely. Additionally, the recent implementation of the EU AI Act9 adds another layer of regulatory compliance, ensuring that AI in Europe is safe, respects fundamental rights, and upholds democracy.
IBM Consulting brings a valuable and responsible approach to AI.
GAI vs AGI
Recently, there has been growing concern regarding the potential confusion between AGI (Artificial General Intelligence) and GAI (General AI). It’s crucial to clearly distinguish between these two concepts. AGI refers to a theoretical form of AI that possesses the ability to understand, learn, and apply intelligence in a wide range of tasks, mirroring human cognitive skills. In contrast, GAI is currently in use in various sectors, including banking as we described in this article, and is designed for specific, narrow tasks. This differentiation is crucial for accurately assessing the risks and regulatory requirements associated with AI technologies. Misunderstanding the capabilities and limitations of each could lead to misinformed discussions and decisions in the field of artificial intelligence. Right now, when we talk about the risks and rules of AI, like those in the EU AI Act, we’re mostly talking about GAI. AGI isn’t something we have yet. Understanding this difference helps us know what risks we’re dealing with and what the current rules are about.
Conclusion
In the ever-changing world of finance, generative AI is truly transformative. As we’ve seen its impact in 2023, gen AI is reshaping the industry in many ways. It’s improving fraud detection, providing 24/7 customer service, enhancing cybersecurity, and offering personalized advice and assistance. However, with these opportunities come important considerations like protecting sensitive data, addressing potential bias, and managing cybersecurity risks. It’s clear that gen AI can be a valuable ally, but its success relies on a careful and responsible approach that prioritizes customer trust, security, and ethical use. The financial world is entering a new era, where gen AI can help navigate the changing landscape.
Acknowledgments
I would like to express my sincere gratitude to those who have made the publication of this article possible. Special thanks to Armin Warda for his expert guidance on the financial sector’s trends and insights, which greatly enhanced the depth of this work. I am also thankful to Matthias Pfützner and Ingo Boernig whose assistance and insights were invaluable in the final stages of this work.
- https://medium.com/@ashusingh19911082/agi-artificial-general-intelligence-are-we-there-yet-2873522cb412 ↩︎
- https://www.altexsoft.com/blog/language-models-gpt/ ↩︎
- https://medium.com/@ashusingh19911082/agi-artificial-general-intelligence-are-we-there-yet-2873522cb412 ↩︎
- GAI is Not a Typo for AGI, ToM is Not Your Friend from Down …linkedin.comhttps://www.linkedin.com › pulse › gai-typo-agi-tom-yo…
↩︎ - https://www.linkedin.com/pulse/generative-ai-applications-next-frontier-fraud-aruna-pattam/
↩︎ - https://fintech.global/2023/05/16/is-the-era-of-robo-advisors-over/
↩︎ - State of Cybersecurity 2023: Navigating Current and … – ISACAisaca.orghttps://www.isaca.org › news-and-trends › isaca-now-blog ↩︎
- https://fintechmagazine.com/articles/nvidia-advancing-cybersecurity-efforts-with-gen-ai ↩︎
- The EU AI Act, recently agreed upon by the European Parliament and Council, establishes comprehensive rules for trustworthy AI. Key provisions include safeguards on general-purpose artificial intelligence, limitations on the use of biometric identification systems by law enforcement, bans on social scoring and AI used to manipulate or exploit user vulnerabilities, and the right of consumers to launch complaints and receive meaningful explanations. The Act also introduces fines for non-compliance, ranging from 35 million euros or 7% of global turnover to 7.5 million euros or 1.5% of turnover. ↩︎